Navigating the AI Revolution – From Prototype to Production
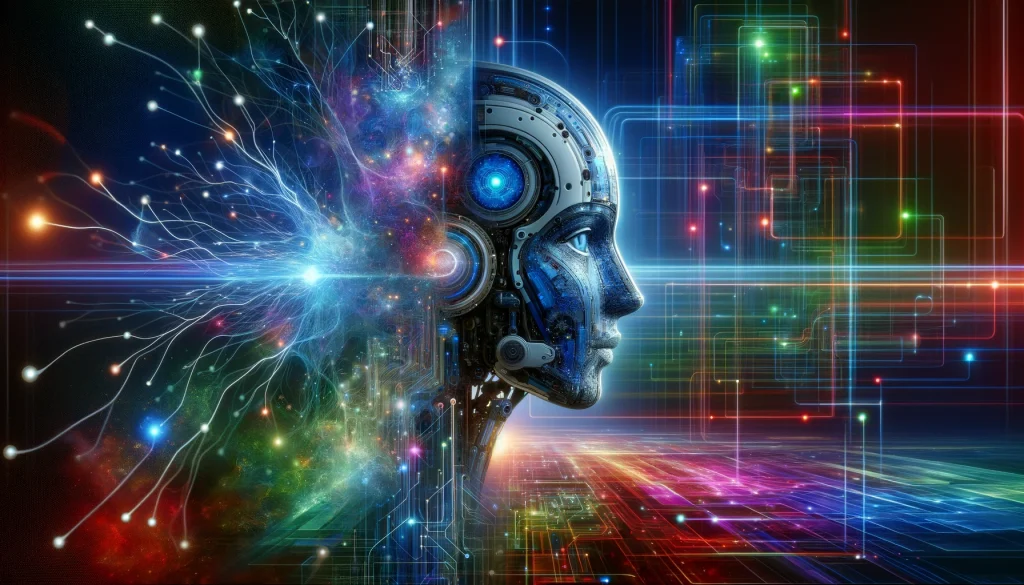
A digital depiction of AI's transformative journey — from the drawing board to the digital frontier.
- What are the recent developments in AI, specifically regarding ChatGPT and GPT-4?
- How has the transition of AI models from experimental tools to integral workflow components occurred?
- What are the key challenges in transitioning AI applications from prototype to production?
- What framework is proposed for scaling AI applications?
- How important is user experience in AI application development, and what strategies should be employed?
- What does model consistency mean in the context of AI, and how can it be achieved?
- Why is evaluating AI application performance crucial, and how can it be effectively done?
- What are the key considerations for managing the scale of AI applications?
- What is LLM Ops, and why is it emerging as a key field in enterprise architecture?
- What is the outlook for AI application development, and how can individuals contribute?
Welcome to the exciting frontier of AI development, where the lines between science fiction and reality blur with every passing day. Today, we’re diving into an enthralling discussion led by Sherwin and Shyamal, two luminaries from OpenAI, who recently illuminated the path from AI prototype to production. If you’re captivated by the swift evolution of AI tools like ChatGPT and GPT-4, this post is your gateway to understanding how these innovations are reshaping our digital landscape.
This blog post synthesizes and discusses concepts presented in an OpenAI video, which can be viewed in full here. For the most comprehensive insights, viewers are encouraged to watch the original video.
The Emergence and Impact of GPT Models
Remember when ChatGPT first waltzed into our lives? It feels like it’s been ages, but this AI marvel made its debut less than a year ago, in late November 2022. Fast forward a few months, and we were introduced to its sophisticated sibling, GPT-4, in March 2023. These AI models have rapidly transitioned from being fancy tech novelties to integral parts of our daily workflow, sparking a revolution in how startups, developers, and enterprises integrate AI into their products.
Transitioning from Prototype to Production: The Challenges
But let’s pump the brakes for a moment. Migrating an AI application from a cool prototype to a fully functional production tool is no walk in the park. This process is riddled with complexities, especially given the unpredictable nature of AI models. This is where our experts, Sherwin and Shyamal, step in with a guiding framework to navigate these choppy waters.
The Proposed Framework: A Stack Diagram Approach
Imagine a stack diagram, a structured blueprint laying out the roadmap for scaling AI applications. This isn’t just any framework; it’s a lifeline for developers embarking on the daunting journey of transforming their AI prototypes into robust production-level applications. The stack is broken down into four key components: User Experience, Model Inconsistency Handling, Evaluation Strategies, and Orchestration for Scale.
Building a Delightful User Experience
When it comes to AI applications, user experience is king. Sherwin and Shyamal emphasize strategies that focus on uncertainty management and safety guardrails. The goal is to create AI-assisted interfaces that are not only intuitive but also secure and trustworthy, ensuring a seamless human-AI interaction.
Ensuring Model Consistency
In the rapidly evolving world of AI, consistency is the golden key to reliability. Sherwin and Shyamal introduce the concept of grounding models with real-world knowledge and tools. They shed light on new model-level features designed to enhance consistency, such as JSON mode and reproducible outputs. These innovations are crucial for developers who seek to tame the inherent unpredictability of AI models, ensuring that their applications respond accurately and reliably across various scenarios.
Evaluating AI Application Performance
No AI application can be deemed successful without thorough evaluation. The duo emphasizes the importance of creating bespoke evaluation suites, tailored specifically to different use cases. This process involves both human judgment and AI-powered assessments, blending the best of both worlds to refine and perfect AI applications. Evaluations serve as a compass, guiding developers through the labyrinth of AI development, ensuring that their creations consistently meet high standards of performance and reliability.
Managing Scale: Cost and Latency Considerations
Scaling an AI application isn’t just about handling more users; it’s about managing resources smartly. Here’s where the concept of orchestration shines. The speakers introduce strategies like semantic caching and routing to cheaper models, which are essential in balancing the scales of performance and cost-efficiency. As AI applications grow, these strategies become vital in maintaining a smooth, responsive service without breaking the bank.
The Emergence of LLM Ops
Enter the realm of Large Language Model Operations (LLM Ops), a nascent field that is fast becoming a cornerstone in enterprise architecture. LLM Ops is akin to a master key, unlocking the full potential of AI applications by streamlining their development and deployment. This discipline focuses on operational management, addressing challenges such as performance optimization, security compliance, and data management. It’s a testament to the complex ecosystem that AI development has become and the need for specialized practices to navigate it effectively.
Conclusion and Future Outlook
As we stand on the cusp of a new era in AI, it’s clear that the journey from prototype to production is laden with challenges, but also brimming with opportunities. The insights shared by Sherwin and Shyamal not only illuminate the path but also invite us to think creatively about the future of AI applications. This is a call to action for innovators, developers, and dreamers to collaborate and push the boundaries of what’s possible with AI.
Are you ready to dive into the world of AI development? We encourage you to explore further, experiment with OpenAI tools, and share your experiences. The future of AI is not just written by a select few; it’s a collaborative canvas waiting for your unique brushstrokes. Join the conversation, share your thoughts, and let’s shape the future of AI together.
Source Acknowledgment
This blog post was inspired by and based on insights from a detailed and informative video discussion. To delve deeper into the topics covered here and to gain further insights from the experts themselves, Sherwin and Shyamal from OpenAI, I highly recommend watching the full video. You can find it on YouTube at “The New Stack and Ops for AI“. This video serves as a valuable resource for anyone interested in the evolving landscape of AI and its practical applications.