AI in the Legal Arena: Hallucinations and the Need for Transparency
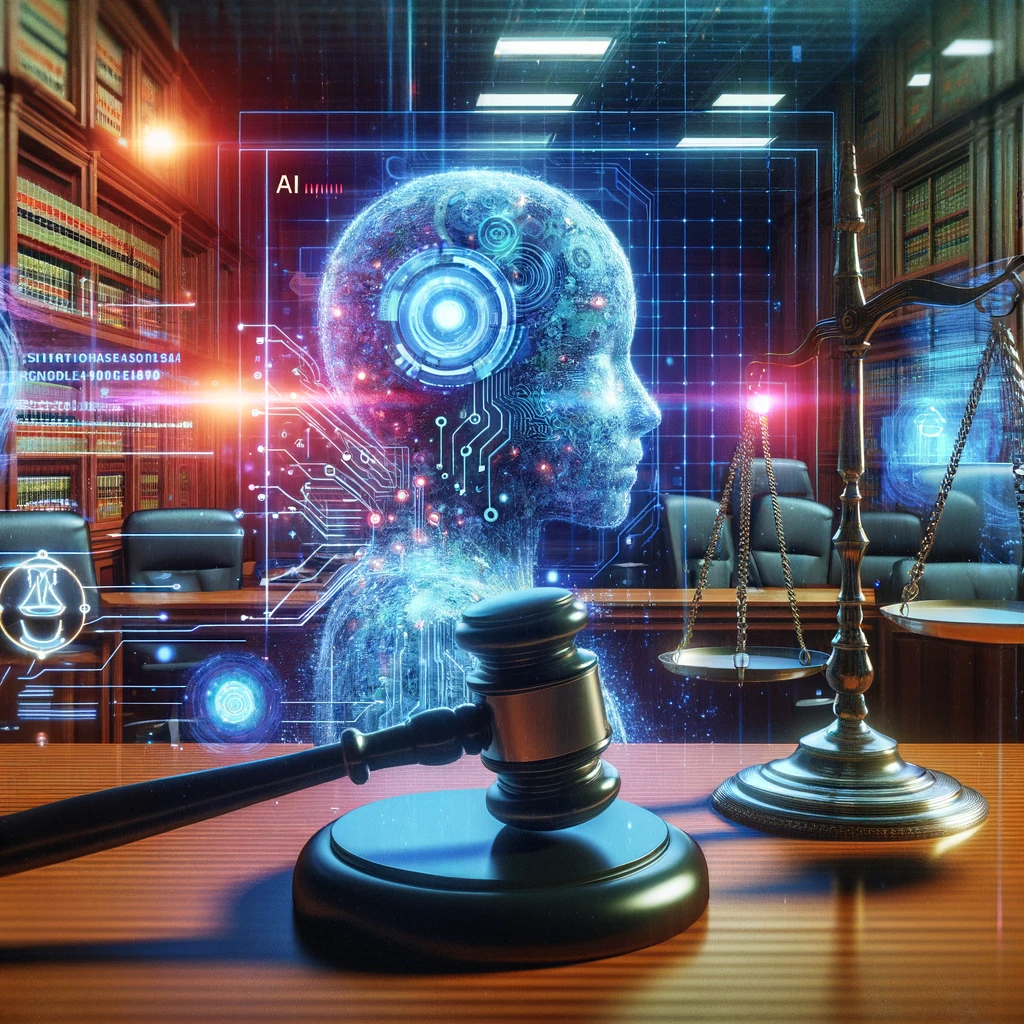
A conceptual illustration showcasing the integration of AI with the legal system, highlighting the challenges and advancements in utilizing AI for legal practice.
- How reliable are AI tools in legal practice, and what challenges do they present?
- What is the significance of hallucinations in AI-generated legal research, and how can they be mitigated?
- Why is transparency and rigorous benchmarking crucial for the responsible integration of AI into legal practice?
Artificial intelligence (AI) is rapidly transforming law practice, promising to streamline various legal processes and improve efficiency. Nearly three-quarters of lawyers plan on using generative AI for their work, from sifting through mountains of case law to drafting contracts to reviewing documents to writing legal memoranda. However, the reliability of these tools remains a critical concern. A new study by Stanford University’s Human-Centered AI (HAI) and Stanford RegLab reveals that legal AI tools still hallucinate—generate false or misleading information—at alarming rates. This finding underscores the urgent need for rigorous benchmarking and public evaluations of AI tools in the legal domain.
The Problem of AI Hallucinations
Large language models (LLMs) like those used in generative AI have a well-documented tendency to hallucinate. These hallucinations can manifest in two ways: producing entirely incorrect information or providing citations that do not support the claims made. In one highly-publicized incident, a New York lawyer faced sanctions for citing fictional cases generated by ChatGPT in a legal brief. Similar cases have been reported, highlighting the risks of incorporating AI into legal practice.
According to the study, general-purpose chatbots hallucinated between 58% and 82% of the time on legal queries. This high error rate has significant implications for the use of AI in law, where accuracy and reliability are paramount. Chief Justice Roberts, in his 2023 annual report on the judiciary, warned lawyers about the dangers of AI hallucinations.
The Promise and Reality of Retrieval-Augmented Generation (RAG)
Retrieval-augmented generation (RAG) is widely promoted as a solution to reduce hallucinations in domain-specific contexts like law. RAG systems integrate a language model with a database of legal documents, aiming to deliver more accurate and trustworthy information. Leading legal research services like LexisNexis (creator of Lexis+ AI) and Thomson Reuters (creator of Westlaw AI-Assisted Research and Ask Practical Law AI) claim that their RAG-based tools can “avoid” hallucinations and guarantee “hallucination-free” legal citations.
However, the study by Stanford RegLab and HAI researchers reveals a different reality. While these bespoke legal AI tools do reduce errors compared to general-purpose models like GPT-4, they still hallucinate significantly. The Lexis+ AI and Ask Practical Law AI systems produced incorrect information more than 17% of the time, while Westlaw’s AI-Assisted Research hallucinated more than 34% of the time.
Understanding the Study and Its Implications
To assess the reliability of these AI tools, the researchers manually constructed a pre-registered dataset of over 200 open-ended legal queries. These queries were designed to probe various aspects of the system’s performance, including general research questions, jurisdiction or time-specific questions, false premise questions, and factual recall questions. The study aimed to reflect a wide range of query types and to constitute a challenging real-world dataset.
The researchers identified two main types of hallucinations: incorrect responses and misgrounded responses. An incorrect response describes the law inaccurately or contains factual errors, while a misgrounded response describes the law correctly but cites sources that do not support the claims made. The latter type of hallucination is particularly concerning because it can mislead users into placing undue trust in the tool’s output, potentially leading to erroneous legal judgments and conclusions.
For example, Westlaw’s AI-Assisted Research product made up a statement in the Federal Rules of Bankruptcy Procedure that does not exist, while LexisNexis’s Lexis+ AI cited a legal standard that had been overturned by the Supreme Court. These errors highlight the potential dangers of relying on AI tools without thorough verification.
The Challenges of RAG Systems in Legal AI
Despite the theoretical advantages of RAG systems, the study identifies several challenges unique to the legal domain that contribute to hallucinations. First, legal retrieval is inherently difficult. The law is not composed solely of verifiable facts but is built up over time by judges writing opinions. Identifying the set of documents that definitively answer a query can be challenging, and retrieval mechanisms may fail.
Second, even when relevant documents are retrieved, they may not be applicable due to differences in jurisdiction or time period. This issue is particularly problematic in areas where the law is in flux. For instance, one system incorrectly recited the “undue burden” standard for abortion restrictions as good law, which was overturned in Dobbs v. Jackson Women’s Health Organization.
Third, the tendency of AI to agree with the user’s incorrect assumptions, known as sycophancy, poses unique risks in legal settings. For example, one system naively agreed with the incorrect premise that Justice Ginsburg dissented in Obergefell v. Hodges, the case establishing a right to same-sex marriage, and provided additional false information.
The Need for Transparency and Benchmarking
The study’s findings highlight the critical need for rigorous and transparent benchmarking of legal AI tools. Unlike other domains, the use of AI in law remains alarmingly opaque. Legal AI tools provide no systematic access, publish few details about their models, and report no evaluation results. This opacity makes it exceedingly challenging for lawyers to procure and acquire AI products.
The absence of rigorous evaluation metrics also threatens lawyers’ ability to comply with ethical and professional responsibility requirements. Bar associations in California, New York, and Florida have all recently released guidance on lawyers’ duty of supervision over work products created with AI tools. More than 25 federal judges have issued standing orders instructing attorneys to disclose or monitor the use of AI in their courtrooms.
Without access to evaluations of the specific tools and transparency around their design, lawyers may find it impossible to comply with these responsibilities. Alternatively, given the high rate of hallucinations, lawyers may find themselves having to verify each and every proposition and citation provided by these tools, undercutting the stated efficiency gains.
Moving Forward: Responsible AI Integration
The study emphasizes that legal hallucinations have not been solved and that the legal profession must turn to public benchmarking and rigorous evaluations of AI tools. Legal AI tools like those from LexisNexis and Thomson Reuters are not the only ones in need of transparency. A slew of startups offer similar products and make similar claims, but they are available on even more restricted bases, making it even more difficult to assess how they function.
The responsible integration of AI into law requires transparency and accountability. Legal professionals need access to evaluations and clear information about how AI tools function to make informed decisions about their use. Public benchmarking can provide a standardized way to assess the reliability and accuracy of these tools, ensuring that they meet the high standards required in legal practice.
In conclusion, while AI has the potential to revolutionize the legal field, its current limitations and the prevalence of hallucinations highlight the need for caution. Legal professionals must demand transparency and rigorous evaluations of AI tools to ensure that they can be used responsibly and effectively. As AI continues to evolve, the legal profession must adapt, balancing the benefits of technology with the need for accuracy and reliability.
For more information, you can read the original article on the Stanford University HAI website here.