Unveiling the Power of AI in Cancer Treatment: Predicting HRD and Platinum Response
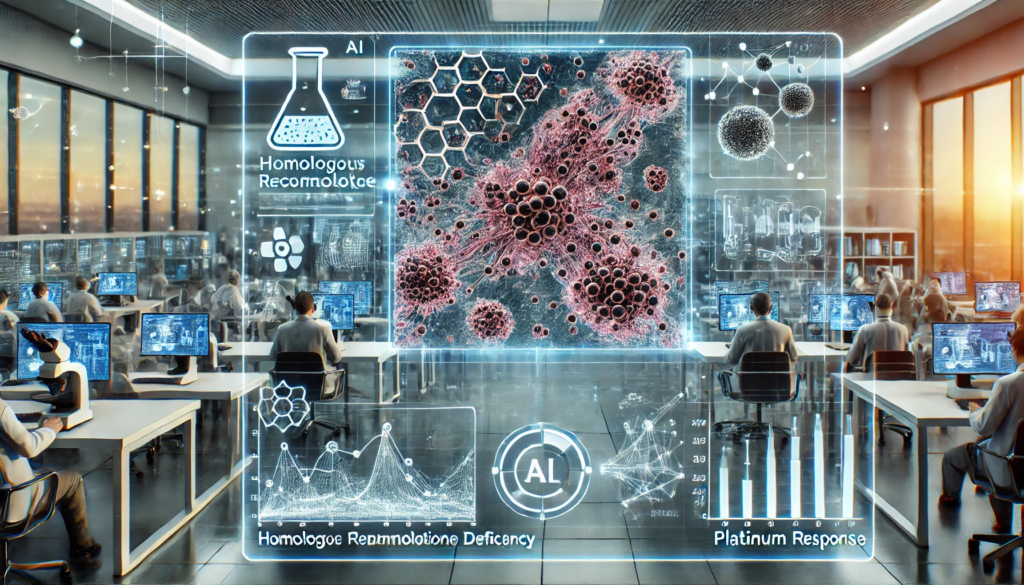
DeepHRD uses AI to predict homologous recombination deficiency and platinum response from routine histopathological slides, offering a revolutionary approach in cancer diagnostics.
- How does DeepHRD use AI to predict homologous recombination deficiency (HRD) from histopathological slides?
- What are the potential benefits of using AI for HRD prediction in cancer treatment?
- How can AI-driven diagnostics like DeepHRD address disparities in cancer care and improve accessibility?
Artificial intelligence continues to revolutionize numerous fields, and healthcare is no exception. A recent study published in the Journal of Clinical Oncology highlights how AI, specifically deep learning, pushes the boundaries of cancer treatment by predicting homologous recombination deficiency (HRD) and response to platinum-based therapies. This groundbreaking research presents significant implications for the future of oncology, potentially transforming patient outcomes and treatment efficiency.
The Study’s Core Findings
The research, conducted by Erik N. Bergstrom and his colleagues, introduces DeepHRD, an advanced AI platform designed to predict HRD directly from routine histopathological slides stained with hematoxylin and eosin (H&E). Traditional methods of detecting HRD rely heavily on molecular profiling, which can be costly, time-consuming, and not universally available. By leveraging AI, the study aims to overcome these barriers and provide a more accessible diagnostic tool.
Performance and Validation
DeepHRD was trained using primary breast and ovarian cancer samples from The Cancer Genome Atlas (TCGA). The AI’s performance was impressive, with an Area Under the Curve (AUC) of 0.81 when predicting HRD from breast cancer slides. This performance was validated in independent cohorts, demonstrating DeepHRD’s robustness across different datasets and conditions. Notably, in a platinum-treated metastatic breast cancer cohort, DeepHRD-predicted HRD samples showed a higher complete response rate and a significant increase in progression-free survival (PFS) compared to traditional methods.
Implications for Clinical Practice
Enhancing Precision Oncology
Precision oncology focuses on tailoring treatments to individual patients based on the specific characteristics of their cancer. The ability to predict HRD using AI significantly enhances this approach. For cancers like breast and ovarian, where HRD indicates a higher likelihood of responding to platinum-based therapies and PARP inhibitors, DeepHRD provides a valuable tool for optimizing treatment plans. This can lead to improved patient outcomes, as those with HRD-positive tumors can be more effectively targeted with appropriate therapies.
Accessibility and Cost-Effectiveness
One of the major advantages of using AI for HRD prediction is the reduction in costs and turnaround times associated with traditional molecular diagnostics. Molecular profiling can cost thousands of dollars per patient and take several weeks to deliver results. In contrast, DeepHRD utilizes digital images of H&E slides, which are routinely prepared in clinical practice. This not only makes the technology more accessible, especially in resource-constrained settings but also accelerates the diagnostic process, allowing for timely treatment decisions.
Broader Impacts and Future Scenarios
Addressing Disparities in Cancer Care
The implementation of AI-driven diagnostics like DeepHRD could help address disparities in cancer care. In many regions, especially low-resource settings, access to advanced molecular diagnostics is limited. By utilizing readily available H&E slides, DeepHRD can provide high-quality diagnostic capabilities to these areas, ensuring that more patients receive the benefits of precision oncology.
Integration with Clinical Workflows
Integrating AI tools into existing clinical workflows poses challenges and opportunities. On one hand, healthcare professionals need to be trained to use and trust these new technologies. On the other hand, AI tools can significantly reduce the workload on pathologists and oncologists, allowing them to focus on more complex aspects of patient care. As AI continues to evolve, its integration into healthcare will likely become more seamless, driven by continuous validation and improvements in AI models.
Ethical and Societal Considerations
Ensuring Ethical AI Use
Strong ethical principles must guide the use of AI in healthcare. Issues such as data privacy, algorithmic bias, and transparency are paramount. Ensuring that AI systems are trained on diverse datasets and are regularly audited for bias is crucial to their fair and effective application. Furthermore, patients should be informed about how AI is used in their care and have the right to consent to its use.
Future of Personalized Medicine
The advancements in AI-driven diagnostics are paving the way for a more personalized approach to medicine. As tools like DeepHRD become more sophisticated, they will enable even more precise targeting of therapies, potentially extending beyond cancer to other complex diseases. This shift towards personalized medicine promises not only to improve outcomes but also to reduce the incidence of side effects by avoiding ineffective treatments.
The research conducted by Bergstrom et al. is a significant milestone in the application of AI in oncology. By predicting HRD from routine histopathological slides, DeepHRD offers a cost-effective, efficient, and widely accessible diagnostic tool. This innovation holds the promise of transforming cancer care, particularly in improving the precision and timeliness of treatment for breast and ovarian cancers. As AI technology continues to advance, its integration into clinical practice will likely drive significant improvements in patient outcomes and the overall efficiency of healthcare systems.
For those interested in the full details of the study, the original article can be accessed here, and a PDF version is available for download here.